Exploring the Future Understanding Crypto Trading Models
المحتويات
- 1 Understanding Crypto Trading Models
- 2 The Basics of Crypto Trading Models
- 3 Types of Trading Models
- 4 1. Fundamental Analysis Models
- 5 2. Technical Analysis Models
- 6 3. Quantitative Trading Models
- 7 4. Sentiment Analysis Models
- 8 5. Arbitrage Models
- 9 6. Machine Learning Models
- 10 Choosing the Right Model
- 11 Common Pitfalls in Crypto Trading Models
- 12 The Future of Crypto Trading Models
- 13 Conclusion
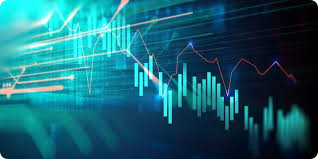
Understanding Crypto Trading Models
The cryptocurrency market, with its volatility and potential for high returns, has captured the attention of investors worldwide. As the landscape continues to evolve, Crypto Trading Models click here to explore the various trading models that have emerged. Understanding these models is crucial for anyone looking to navigate this complex environment effectively.
The Basics of Crypto Trading Models
Crypto trading models refer to the structured approaches traders use to make decisions in the cryptocurrency markets. These models can range from simple techniques like trend following to more complex algorithms that incorporate statistical analysis, machine learning, and artificial intelligence. Understanding these various models can help traders identify which strategies may work best for their specific goals and risk thresholds.
Types of Trading Models
There are several different types of trading models in the cryptocurrency space. Here are some of the most prominent:
1. Fundamental Analysis Models
Fundamental analysis involves evaluating a cryptocurrency’s intrinsic value by examining related economic, financial, and other qualitative and quantitative factors. Traders look at metrics such as developer activity, partnerships, market demand, and overall technology improvements to inform their trades. Popular tools include CoinMarketCap and various blockchain explorers.
2. Technical Analysis Models
Technical analysis is one of the most widely used trading models and involves analyzing statistical trends from trading activity. Traders use price charts and other tools to identify patterns and price movements. Key elements include indicators like moving averages, Relative Strength Index (RSI), and Fibonacci retracement levels. Technical analysts believe that price movements are not random and can be predicted through these methods.
3. Quantitative Trading Models
Quantitative trading applies mathematical computations and statistical techniques to identify trading opportunities. Traders using this model often rely on complex algorithms and automated trading systems. These strategies can analyze vast amounts of data quickly, recognizing patterns and executing trades faster than human traders can.
4. Sentiment Analysis Models
Sentiment analysis involves gauging the mood of the market by analyzing news articles, social media, and other public sentiment sources. Traders utilize sentiment data to anticipate future price movements based on public perception of the cryptocurrency market. It highlights the psychological factors that can drive prices up or down.
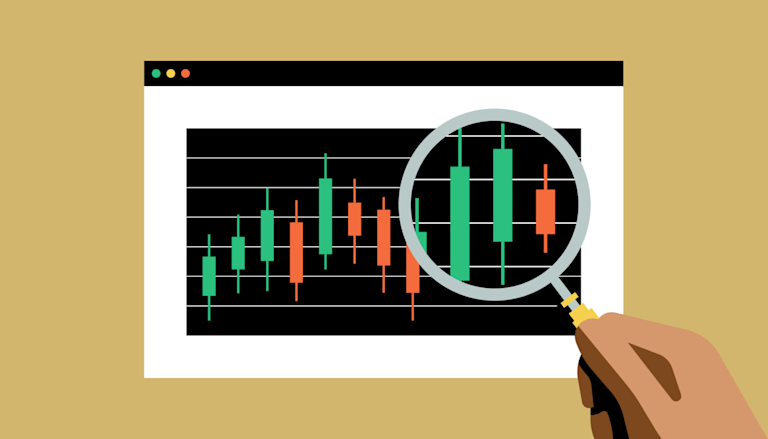
5. Arbitrage Models
Arbitrage trading entails taking advantage of price discrepancies between different markets or exchanges. Traders buy a cryptocurrency at a lower price on one exchange and sell it at a higher price on another. This model relies on the efficiency of the markets, and while it can provide quick profits, it requires significant speed and quick decision-making.
6. Machine Learning Models
With advancements in technology, machine learning models have emerged as a new frontier in crypto trading. These models utilize historical data to make predictions about future price movements. By training algorithms on vast datasets, traders can develop predictive models that adapt over time, optimizing their strategies based on changing market conditions.
Choosing the Right Model
The choice of which trading model to adopt can depend on several factors, including the trader’s risk appetite, investment goals, experience level, and available resources. Beginners may prefer simpler models such as fundamental or technical analysis, while experienced traders looking for higher returns may lean towards quantitative or algorithmic strategies.
Common Pitfalls in Crypto Trading Models
While various trading models can enhance the decision-making process in crypto trading, they are not foolproof. Here are some common pitfalls traders may face:
- Over-Reliance on Historical Data: Many models rely heavily on past data to predict future outcomes. This can lead to significant losses if market conditions change unexpectedly.
- Emotional Decision-Making: Traders can sometimes override model predictions with personal beliefs or emotional reactions, leading to poor outcomes.
- Ignoring Market Conditions: External factors such as regulatory changes or macroeconomic trends can dramatically impact the cryptocurrency market, which may not be accounted for in some models.
The Future of Crypto Trading Models
As the cryptocurrency market continues to evolve, so too will the trading models used by investors. The rise of more sophisticated technology, including artificial intelligence and blockchain analytics, will enable traders to refine their strategies and adapt to the fast-paced market environment.
Moreover, as more institutional investors enter the crypto space, the need for robust, reliable trading models will grow. This will likely bring more standardization and regulation to the market, impacting how trading models are developed and deployed.
Conclusion
In conclusion, understanding crypto trading models is vital for anyone looking to succeed in the cryptocurrency market. From fundamental and technical analysis to advanced machine learning techniques, various approaches can help traders make informed decisions. However, recognizing the limitations of each model and continuously adapting to changing market dynamics is equally important. As technology and market conditions advance, traders must remain agile and open to new strategies to capitalize on the opportunities within this exciting space.